Refining wind power forecasts
A joint study in Europe seeks to improve confidence in forecasts generated by artificial intelligence (AI) models.
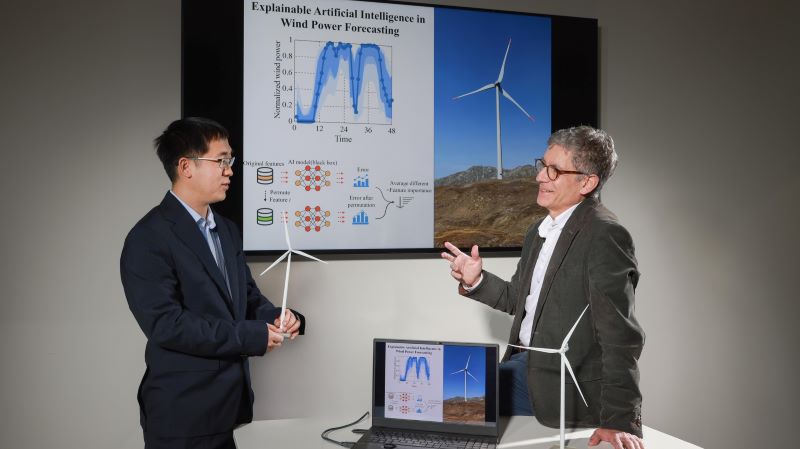
The researchers and study's co-authors Wenlong Liao and Fernando Porté-Agel.
© 2025 EPFL/Alain Herzog - CC-BY-SA 4.0The research applies techniques from 'explainable AI' (XAI) - a branch of AI that helps users look inside models to understand how their output is generated and whether their forecasts can be trusted.
On this occasion, researchers at EPFL’s Wind Engineering and Renewable Energy Laboratory (WiRE) have tailored XAI to the black-box AI models used in their field.
In the study, they found that XAI can improve the interpretability of wind-power forecasting by providing insight into the string of decisions made by a black-box model and help identify which variables should be used in a model’s input.
‘Before grid operators can effectively integrate wind power into their smart grids, they need reliable daily forecasts of wind-energy generation with a low margin of error,’ says Professor Fernando Porté-Agel, head of WiRE.
‘Inaccurate forecasts mean grid operators have to compensate at the last minute, often using more expensive fossil fuel-based energy.’
The models currently used to forecast wind power output are based on fluid dynamics, weather modelling, and statistical methods – yet they still have a non-negligible margin of error.
AI has enabled engineers to improve wind power predictions by using extensive data to identify patterns between weather model variables and wind turbine power output.
XAI provides transparency on the modelling processes.