Machine learning in ceramics & glasses
View this on-demand webinar which took place on Tuesday 29 March 2022.
Please login or create your My IOM3 Account to access this on-demand webinar.
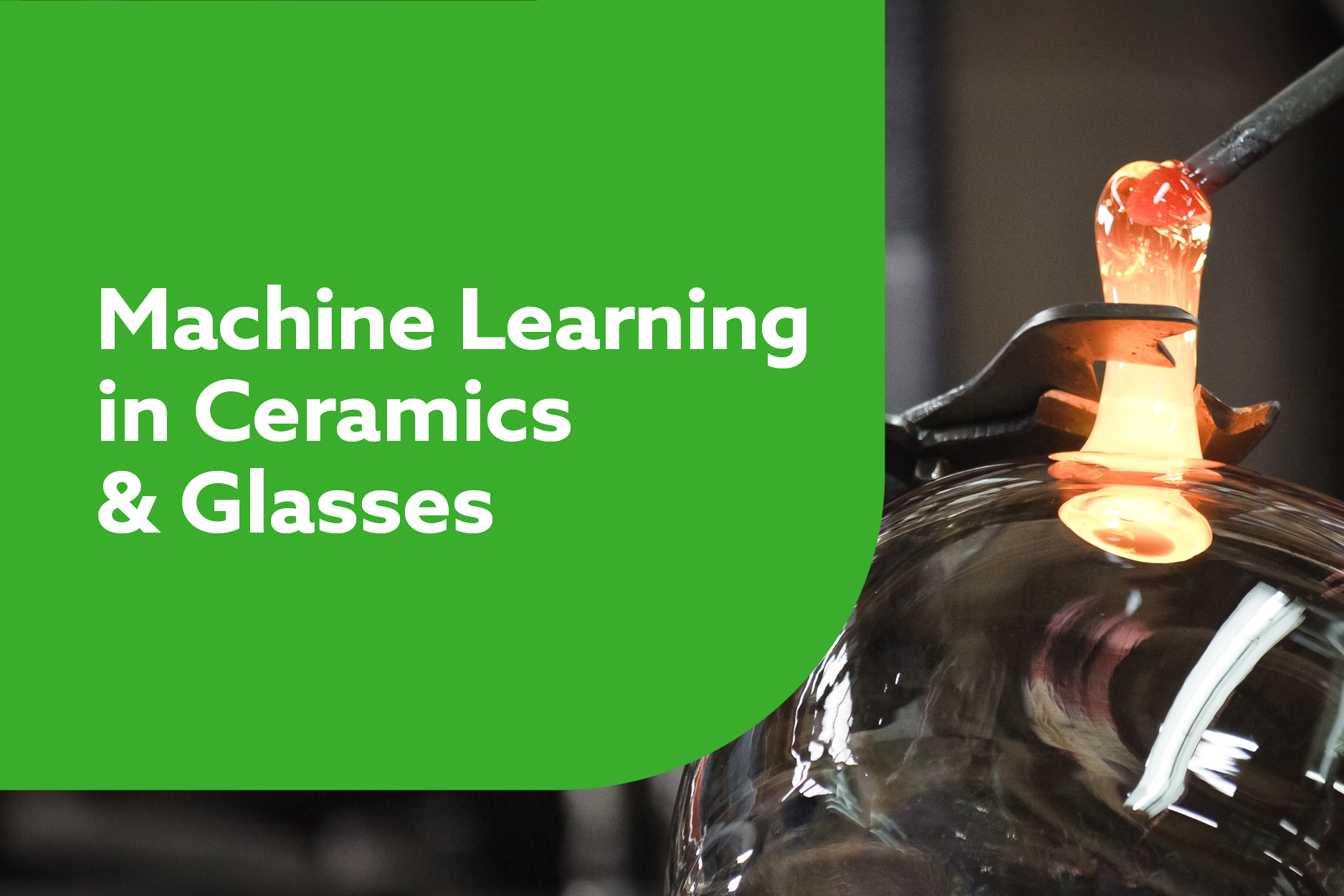
This webinar is focused on the use of machine learning in the area of ceramics and glasses and therefore will have an impact on academics working in the area and on industry.
Three important take away benefits for participants:
- Fundamental knowledge on the use of machine learning in the discovery and design of ceramics/glasses
- Understanding digitalization in ceramic/glass industry
- Knowledge about the development of machine learning the ceramic/glass field in UK
First-principles and machine learning modelling of ceramic materials: the case of AsTe3 thermoelectric material
Presented by Assil Bouzid, Researcher, CNRS
The search for high performing thermoelectric materials led recently to the development of a new crystalline compound with composition AsTe3, that exhibits a very low thermal conductivity and an interesting figure of merit (ZT) peak value of 0.3 at 405 K, indicative of its thermoelectric performance. Nevertheless, the atomic-scale structure of both crystalline and amorphous AsTe3 remain unknown which hinders further improvement of the properties of this compound. In this talk, Assil will show how first-principles molecular dynamics can be combined to machine-learning interatomic potentials to achieve an accurate determination of the atomic-scale structure of this ceramic material. A particular focus will be put on the use of machine learning to extend time- and size-scales beyond those achieved solely by resorting to state-of-the-art ab-initio modelling. Finally, they will show an example of high-throughput calculation used to determine the previously unknown structure of the crystalline phase of AsTe3.
Formation Descriptors for High-Entropy High-Hardness Metal Carbides
Presented by Corey Oses, Postdoctoral Fellow, Duke University
Disorder is both intrinsic to all materials, and also a route to enhanced functionality. A combination of 1) high chemical disorder and 2) metal-nonmetal
bonding offers a new direction for thermal protection materials, wear-resistant coatings, batteries, and catalysts. Engineering these materials for applications demands an understanding of the mechanisms governing synthesizability and other entropy-driven behavior, a challenging task often resolved within a limited context or neglected altogether. A simple modeling paradigm is presented that has already led to the discovery of new metal-carbide systems achieving high hardness values as predicted. Additional factors within the framework are being considered to address the delicate balance between entropy maximization and induced enthalpic penalties that become important in other ceramic systems.
Artificial Intelligence for materials design: advances and remaining challenges
Presented by Adama Tandia, Senior Research Associate, Corning Incorporated
Use of artificial intelligence with materials data sets has significantly improved the speed of discovery of new materials with improved attributes. In the field of glass and polymer design, combinations of different regression methods, such as genetic algorithm, Gaussian processes, and neural networks, and data generation methods, such as molecular dynamics and density functional theory, has led to robust design methods, and near defect free manufacturing processes. Yet, despite the many successes, many serious challenges for faster and more robust algorithms remain before the design and manufacture of materials with optimal characteristics.
Machine-learning correlations between structure and dynamics in supercooled liquids and glasses
Presented by Dr Gerhard Jung, PostDoc, CNRS
One of the most fundamental problems in the field of glass physics is understanding the connection between amorphous, microscopic structure and structural relaxation. While for crystals it is well know that relaxation is induced by well-defined `defects' in the ordered structure, finding an analogous definition for `amorphous defects' in glasses remains to be a great challenge. Many physically-motivated structural descriptors have been suggested to predict dynamical properties from structure with good success but it could be shown in recent years that machine-learned descriptors have the potential to be far superior.
In my talk, I will give a brief summary on different machine-learning technique that have recently been applied to study the physics of the glass transition. Based on this summary, I will discuss open challenges and potential machine-learning approaches which we are currently pursuing to work towards better characterizing structural relaxation in amorphous materials and quantifying its correlation to the microscopic structure.
Speakers
Agenda
13:00 |
Welcome and Introduction |
Dr Sergio Gonzalez Sanchez University of Northumbria |
|||
13:05 |
First-principles and machine learning modelling of ceramic materials: the case of AsTe3 thermoelectric material |
Assil Bouzid Researcher CNRS |
|||
13:25 |
Formation descriptors for High-Entropy High-Hardness Metal Carbides |
Corey Oses Postdoctoral Fellow Duke University |
|||
|
Artificial Intelligence for materials design: advances and remaining challenges |
Adama Tandia Senior Research Associate Corning Incorporated |
|||
14:05 |
Machine-learning correlations between structure and dynamics in supercooled liquids and glasses |
Dr Gerhard Jung PostDoc CNRS |
|||
14:25 |
Closing remarks |
Dr Sergio Gonzalez Sanchez University of Northumbria |
|||
14:30 |
Close |