Digital twins for high-value components
Digital twin usage is growing apace. A group of researchers at the UK’s Advanced Forming Research Centre and the University of Sheffield explore its role in forging high-value components.
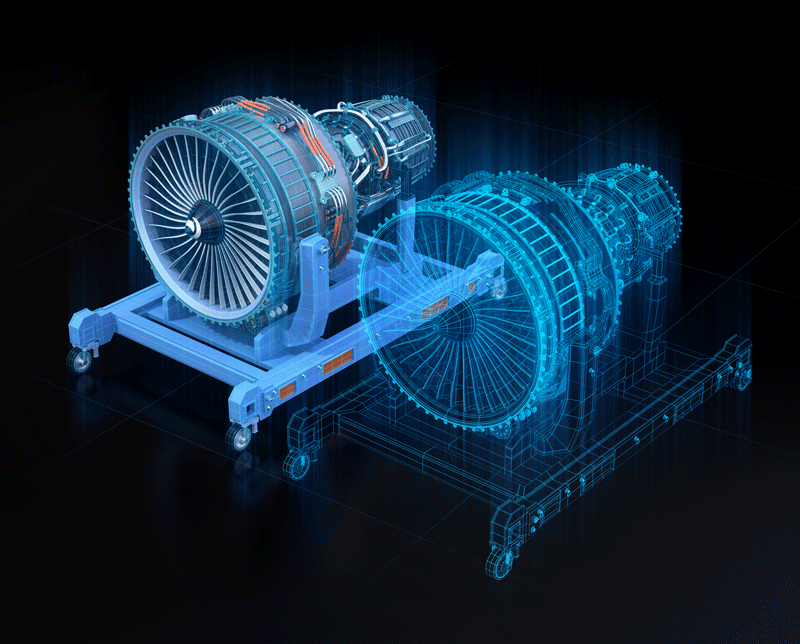
This article was written by Materials Team Lead, Dr Salaheddin Rahimi FIMMM; Computational Materials and Processing Modelling Theme Lead, Dr Ioannis Violatos; and Research Director, Professor Bradley Wynne at the University of Strathclyde’s Advanced Forming Research Centre, UK, a specialist technology centre within the National Manufacturing Institute Scotland, UK; and Professor of Advanced Materials Processing, Professor Martin Jackson, at the University of Sheffield, UK.
Aerospace alloys such as those based on titanium and nickel are produced from their metallic ores through energy intensive reduction and alloying processes. They are then converted to state-of-the-art high-value engineering components by subjecting the material to energy intensive, complex, non-linear, thermo-mechanical processing (forging), which results in heterogeneous microstructure, non-uniform mechanical properties, part distortion and residual stress.
This necessitates significantly larger dimensions than the final geometry to be manufactured, with over 70% of the material then machined away to achieve the final required shape and retain the optimum microstructure and property set necessary for in-service performance.
This expensive and wasteful approach has led to a sector-wide effort to produce components with more homogeneous microstructures and property distributions from less material, with emerging powder-derived manufacturing routes having been explored extensively over recent years.
Emerging manufacturing techniques, such as precision investment casting and additive manufacturing, have advantages over forging in terms of material and energy usage and speed of manufacture, but they cannot produce the high integrity properties required for many structure-critical applications. For now, forging is here to stay, but it needs to have a modern makeover to make it more agile, economical and less wasteful with better performing products.
Forging a digital twin
Despite decades of experience and continuous improvement, forging operations still lack the precise control and tailored production that Manufacturing 4.0 requires. Therefore, for high-integrity products there is enough drive and justification to create a digital twin of the physical forging process to provide a more efficient, less conservative and affordable process route, as well as improved and more consistent properties to reduce design conservatism.
Recent improvements in control, sensor technology and non-destructive testing (NDT) characterisation methods, coupled with improved physical understanding and modelling of the material behaviours and an upturn in computation power, means a digital twin of forging could now be realised. However, it is only achievable through new and data-centric approaches that combine knowledge and know-how of materials behaviour, simulation and modelling with sensing, data analysis and optimisation, and most importantly artificial intelligence (AI) with decision-making capability.
But how achievable and realistic is a digital twin, particularly at this stage where our understanding of micro-scale deformation mechanisms in materials (crystal plasticity) during forging at different temperatures is limited? And even the implementation of existing understanding in crystal-plasticity-based models is limited to small sizes that are far smaller than industrial-scale forging, and so computationally intensive that it cannot be used in process in real time.
A digital twin that incorporates press dynamics, temperature, load, tool wear and lubrication, as well as through-process microstructure and property evolution, will be the closest analogy to an equivalent real-world system or physical twin.
This is not limited to simple animation and parallel simulation of forging processes, but includes robust, multi-scale, physical-based predictive capabilities, in-process and real-time sensing, and fast data optimisation algorithms embedded in a virtual reality framework, with the ability for decision making based on the results of simulations and real-time sensing.
Did you know?
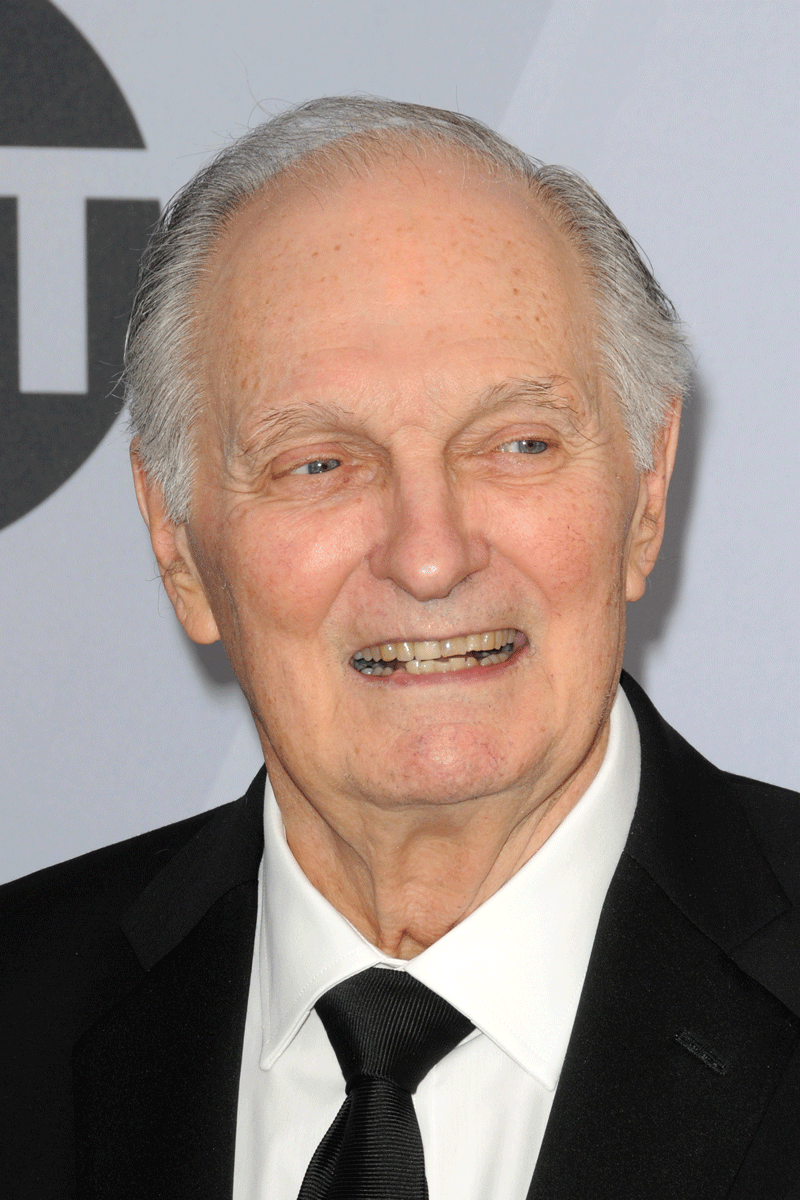
The first mention of the phrase ‘digital twin’ was in 1998 referring to a digital counterpart of American actor Alan Alda.
Alda worked with a US computer animation firm, Lamb & Company, to create a computer model of the actor’s head, made up of around 12,000 contiguous polygons. The final footage involved 2,500 frames (a total of less than two minutes) and took the team several painstaking months to synthesise.
To bring Alda’s speech to ‘life’, the researchers had to correlate the virtual Alda’s lips with simulated audio. ‘We watched how he moves his mouth as he makes an ‘oh’ or ‘ooh’ sound,’ Jim Russell, Technical Director on the project told Scientific American in 1998. ‘We tried to get a feel for how his face should move.’
Twin types
Creating a microstructure digital twin is rather more difficult than a process digital twin. This is because there is currently no NDT method for real-time, in-process, microstructure characterisation, and we cannot implement sensors in the microstructure during the process. Therefore, we must rely on physically based models and post-mortem characterisations. These physical models are complex, time-consuming and computationally expensive, but approaches exist for identifying relevant data from regions of interest.
The process digital twin, meanwhile, relies on shape changes, temperature gradients, loads, deformation rate, etc. from the physical twin. The two digital twins are inter-related with close interactions, and feed to one another.
Inevitably, questions arise as to whether the digital twin is, (1) a set of carefully statistically validated simulation models for a particular process/part; (2) a set of data collected before, during or after forging for the process and the product; (3) a set of real-time simulation/predictive/optimisation tools for engineering decision making, which are built from huge datasets. These are fundamentally different approaches to create a digital twin and each has its own limitations.
The first is based on high fidelity modelling and statistical calibration, which is still not possible to exercise with live data due to limitations in computational power and idealisation of the process itself.
The second relies on collecting all data for the necessary key process parameters, which is practically impossible for most forging processes, and then controlling the process based on a baseline design or manufacturing curve.
The third is more in the realms of AI and fast surrogates, such as deep-learning and neural networks, which operate blindly on large data. It is also difficult and usually expensive to generate, without making use of the physics and dynamics involved in the process.
A digital twin of forging, with real-time decision-making ability, is actually making use of all three approaches and combines them into a more complete and robust tool, complementing each other to extend their limitations.
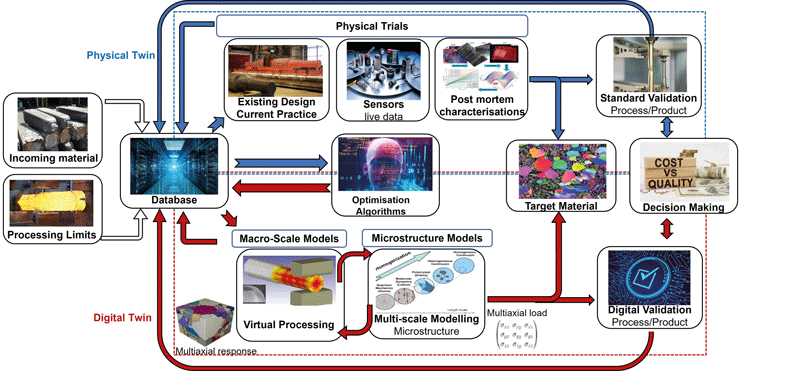
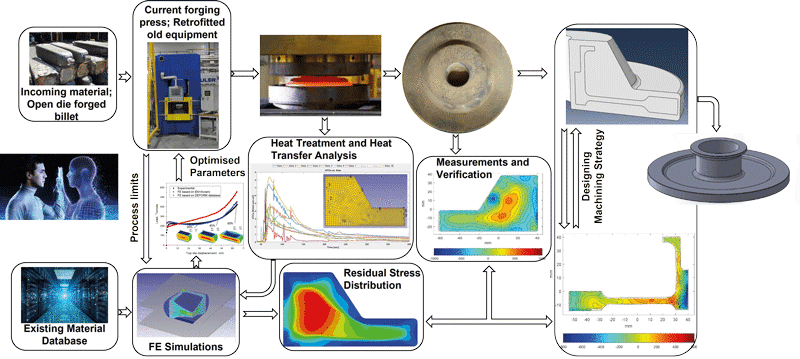
In the frame
Noting the limitations with existing technologies, a microstructurally enabled digital twin of forging can be developed centred on linking high-fidelity spatially resolved full-field models for microstructure evolution and deformation with computationally efficient mean-field models using a multi-level statistical framework.
The full-field models are based on advanced techniques that combine the multi-phase-field method and anisotropic crystal plasticity within a multi-physics simulation framework. These models incorporate effects of phase transformation, deformation and annealing during deformation, cooling and heating, as well as transient effects. They are calibrated using a suite of characterisation techniques, such as laboratory simulation, in situ synchrotron and neutron diffraction, as well as high-resolution electron backscatter diffraction (EBSD).
Mean-field models employ homogenisation approaches to implicitly describe the microstructure and its evolution through crystal-plasticity-based equations operating on a statistically equivalent material, with average characteristics like grain size, dislocation density and texture. This high-fidelity simulation capability can be used to generate virtual datasets to supplement the limited/expensive experimental data required to calibrate the microstructure digital twin (see image above).
For example, consider a simple machining operation on an as-forged and heat-treated high-value disc component for application in jet engines (see image opposite). A baseline model will be developed simulating the metal forging and heat treatment processes – hot working, ageing, quenching, etc. – and taking into consideration microstructural changes such as recrystallisation, precipitations, grain growth, etc., using physically based and constitutive materials models.
A baseline model will also be developed for the subsequent machining operation – metal removal rate, heat generated, etc. – using simplified approaches for metal cutting through material removal operations and integrating cutting forces, machining induced effects, and clamping configurations.
These baseline models are then adapted to create a digital twin that also estimates microstructure changes, generation and evolution of residual stress, and distortion throughout the whole processes. The digital twin will be brought to life using data acquired by in situ measurements where the model is repeatedly updated, improved by learning algorithms, and validated using sensors transmitting real-time data – for example, using data-analytic processed deformation data from digital image correlation.
The dynamic digital representation of the forging process will also lend itself to augmented reality overlay communication tools, enabling engineers to understand, predict, control and optimise the performance of the manufacturing route.
This will be built upon to develop a more complex digital twin representing sequential fabrication operations, proof testing, quality control checks and component lifetime performance. This digital-based technology has the potential to transform the design, manufacture and performance of high-value products, reducing asset downtime, improving productivity and increasing market agility.
This will inform industry on the optimum processing route for a given component, enable assessment of the process route, and allow the whole supply chain to be involved in the early stages of component design, when changes can still be made cheaply through the virtual digital twin. Also, it will enable real-time production decisions, instant troubleshooting and validation for future high-integrity forged components, using physics-based models and data analytics.
From a sustainability standpoint, a digital twin of the microstructure during forging and press performance will enable the supply chain to do more with less material by providing higher confidence in location-specific properties or using the press more efficiently – therein using less energy – to achieve the property goals of the design.
Step-by-step
How can this rather sophisticated technology, which requires high-tech infrastructure and highly trained engineers, be adopted by the forging industry, most of whom rely on expensive legacy equipment with limited adaptability? Most manufacturers, especially SMEs, cannot afford scrapping their legacy equipment for the sake of digital transformation. But it is possible to retrofit them with data acquisition modules, and maybe even new control systems, to obtain/record some critical parameters (such as load and temperature), that can be used as input to the digital twin. Some other necessary parameters can be obtained from historical data or verified simulation models.
Of course, the interactions between the digital twin and legacy equipment may not be fully automatic, but optimised process parameters obtained from a verified digital twin can be implemented into conventional forging processes manually. This is a step towards digitally informed forging to achieve a part with certain microstructural characteristics and mechanical properties set.