Automating materials design
A patent for automating materials design using data mining.
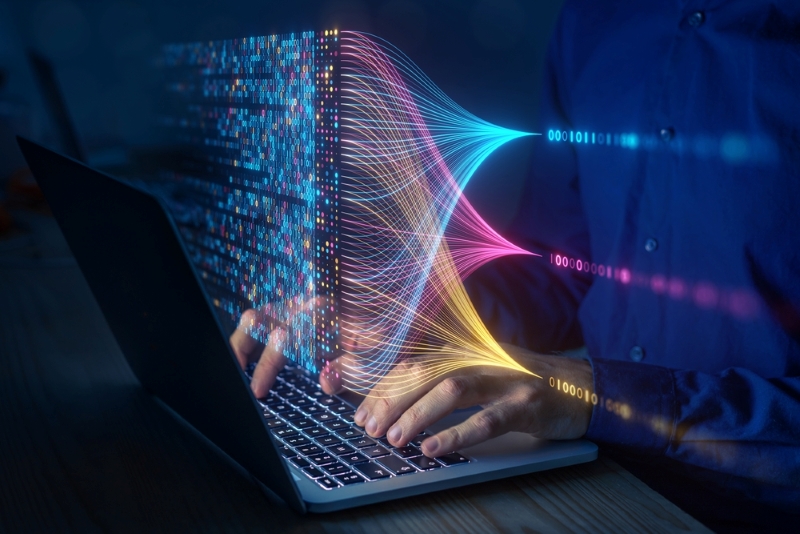
Materials selection and design, targeted at specific properties for particular technical applications, can be time-consuming and expensive. For example, alloy design typically involves equilibrium phase diagrams to predict likely suitable candidate compositions, experimental synthesis of samples, and subsequent analysis using compositional and microstructural characterisation.
A theoretical understanding of the physicochemical relationship between material properties and the composition or structure is also often necessary to guide materials design. Moreover, technicians require the knowledge and skills to interpretate computational and experimental data.
It is therefore not surprising that many companies and research groups are turning to artificial intelligence and other computational methods to automate, simplify, or indeed, improve materials design.
An example is set out in European patent EP3055802 – Methods of selecting material compositions and designing materials having a target property – which was granted to Oerlikon Metco US Inc in June 2023. The patent claims protection for a computer-implemented method of selecting an alloy composition with a target property.
The method begins with receiving an input comprising thermodynamic phase data for a plurality of alloys, where each alloy is a multiphase system with at least four elements. The thermodynamic phase data for each of the plurality of alloys is calculated by varying an amount of each of three or more of the alloying elements simultaneously over a specified composition range. The thermodynamic phase data can include phase diagram data calculated, for example, using the CALPHAD method.
A plurality of thermodynamic quantities corresponding to each of the alloys is then extracted from the thermodynamic phase data and stored. These quantities have been experimentally predetermined to be correlated to microstructures associated with the desired physical properties of the alloy. Examples of suitable quantities include the mole fraction of a particular material phase at a given temperature, a formation temperature of a material phase, a dissolution temperature of a material phase, or a transition temperature between two phases, among others.
Information associated with a ranked subset of the alloys is output, as a plurality of data points plotted in a 2D or 3D graphical image with at least two of the thermodynamic quantities represented as at least two of the axes, where each of the data points represents an alloy composition.
The stored thermodynamic quantities are then mined to rank at least a subset of the alloys based on a simultaneous comparison of two or more thermodynamic quantities that are correlated to the target property. Finally, the composition is selected from a ranked subset of the alloys.
The patent includes four examples illustrating implementations of this method.
In example one, the target is a hard-banding alloy composition that is non-magnetic and possesses both high wear resistance and hardness. The inventors explain that identifying an iron-based alloy combining these properties is difficult because the non-magnetic, face-centred cubic (FCC), polymorph austenite is also the softest phase.
A combination of experimentation and computer modelling had previously identified that (i) a minimum FCC to body-centred cubic (BCC) transition temperature of 950K and (ii) a minimum hard particle phase fraction of 20mol % at 1,300K are thermodynamic criteria useful for ensuring that alloys have a high probability of meeting the desired performance requirements.
Thermodynamic criterion (i) is perhaps counterintuitive because it suggests selecting an alloy that is, according to the phase diagram, magnetic at room temperature. However, the inventors explain that the phase diagram does not itself always contain the information useful for alloy design – the most useful thermodynamic quantities may be counter to conventional metallurgical assumptions.
In the example, 4,408 alloy compositions were evaluated initially and the relevant thermodynamic quantities were extracted from the generated phase diagram data. The results were then filtered down to 643 alloys meeting the minimum requirements for criteria (i) and (ii). The filtered subset was then ranked by hard-particle phase fraction to produce a list of five compositions likely to produce the hardest and most wear-resistant non-magnetic alloys.
The other examples provided in the patent focus on selecting iron-based compositions with different combinations of properties.
In example two, the target is a crack-resistant, hard-facing alloy that combines both high hardness and high toughness. In example three, the target is an abrasion and corrosion-resistant hard-facing alloy. In example four, the target is a crack-resistant, non-magnetic, hard-banding alloy.
Examples three and four involved mining datasets generated in examples one and two for 15,000 different alloys. This meant that the amount of time required for the overall process in examples three and four could be reduced by a factor of about 15, as compared to generating all the necessary data from scratch.
The inventors explain that once the necessary thermodynamic phase data has been generated and stored, the data can be mined repeatedly and used to design alloys for a variety of different desired microstructural and performance criteria.