Machine learning in metallurgy
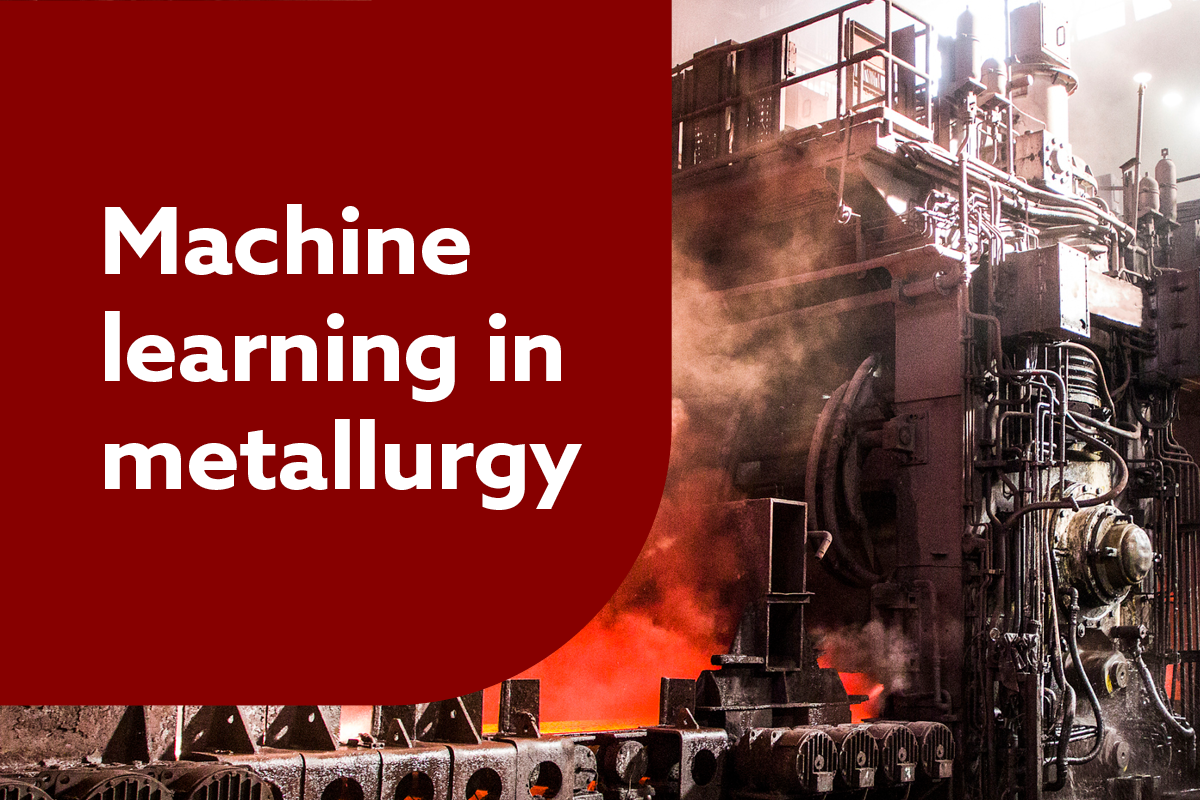
Machine learning in metallurgy is increasingly becoming of interest by organisations to aid discovery and design of metallic materials. Featuring presentations from academia and industry, this webinar is focused on understanding the use of machine learning in metallurgy, it’s impact on industry and the development of machine learning in the UK.