Machine Learning in Electron Microscopy Towards the Attosecond Resolution
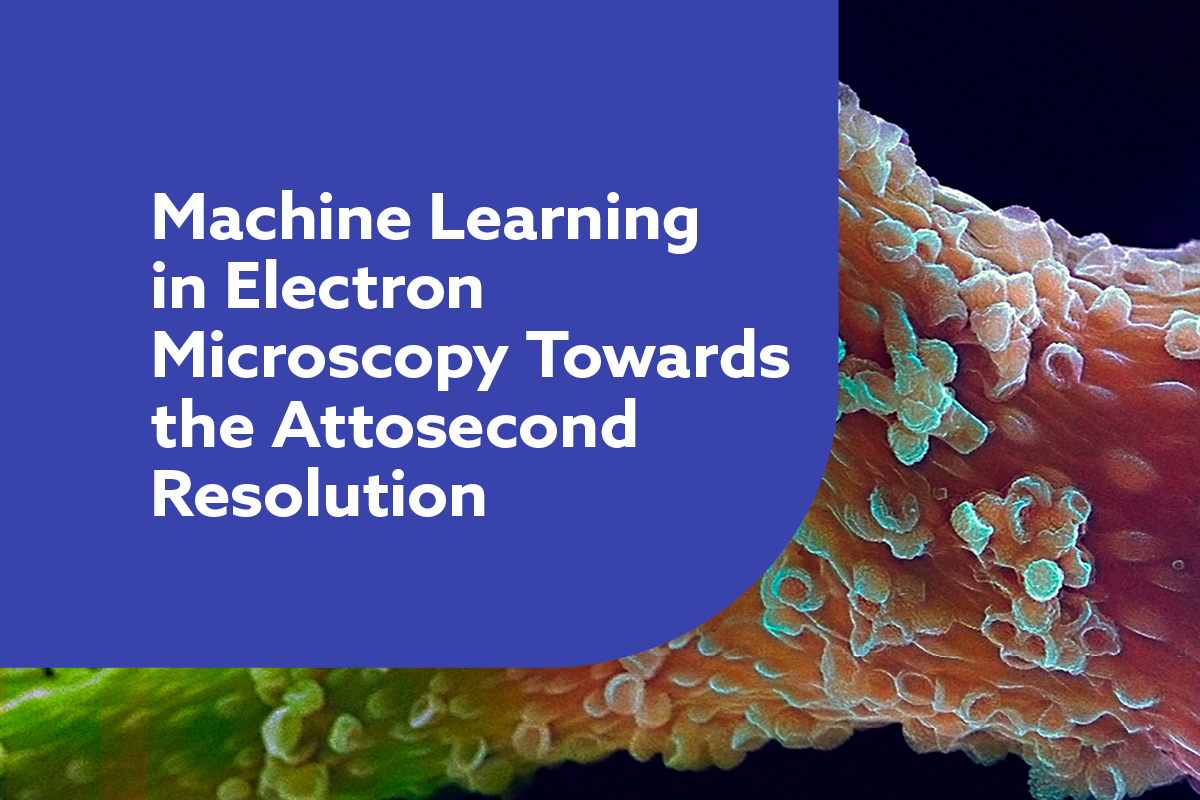
This webinar is focused on the use of machine learning in the area of matter by focusing in microscopy.
Join us for a groundbreaking webinar exploring the future of materials science! This is the first event of its kind, focusing on how machine learning can be applied to attosecond electron microscopy to revolutionise our understanding and control of electron motion dynamics in matter.
As we move to smaller scales, predicting and designing the performance of materials becomes increasingly complex, making machine learning an essential tool. Don’t miss this opportunity to delve into cutting-edge research and innovation at the intersection of machine learning and materials science!
If you would like to take part in this event as a speaker, reach out to James Lee.
3 key takeaways:
- The opportunity to brainstorm for the first time the infinite possibilities that could bring machine learning in attosecond electron microscopy and diffraction
- Dr. Mohammed Th. Hassan from the University of Arizona to talk about his technology: The world’s fastest microscope makes its debut
- Knowledge about the field and potential future investment and development of this area in UK
Agenda |
15-minute presentations
15:00 | Welcome Introduction by Sergio Gonzalez Sanchez |
15:05 |
Revolutionising Electron Microscopy: The Impact of Machine Learning on Nanoscale Imaging and Analysis |
15:25 |
Benchmarking of Additive Manufacturing Machines: A Standards-Based Approach |
15:45 |
Attosecond electron microscopy and diffraction |
16:05 |
Machine Learning-Enabled Image Classification for Automated Electron Microscopy Alexandra Day, Northwestern University |
16:30 | Close of webinar |
|
Abstracts |
Dr Mohammed Th. Hassan - Attosecond electron microscopy and diffractionUltrafast Electron Diffraction and Microscopy imaging have been demonstrated to be pivot tools for imaging the atomic motion in real-time and space 1-3. The generation of a few hundred femtoseconds electron pulses enabled recording movies for molecular and atomic motion4. However, the technical challenges in electron pulse compression have limited the temporal resolution of electron imaging experiments to a hundred femtoseconds. Here, we demonstrate the attosecond temporal resolution in the transmission electron microscope by optical gating 5 to establish what we so-called “attomicroscope” 6. Moreover, we utilized the attomicroscope to image the electron motion dynamics in graphene. In a strong field, the electron is moving in the reciprocal space following the waveform of the driver field. The attosecond electron diffraction experiment allowed us to study the electron density distribution in the reciprocal space at different time instants and connect it with the electron motion in real space. The demonstrated attomicroscopy imaging tool opens the avenue to study electron motion in neutral matter and promises new electron imaging applications in physics, chemistry, and biochemistry 6.
Adv.10,eadp5805(2024). Dr Marc Botifoll - Revolutionising Electron Microscopy: The Impact of Machine Learning on Nanoscale Imaging and AnalysisRevolutionising Electron Microscopy: The Impact of Machine Learning on Nanoscale Imaging and Analysis In recent years, electron microscopy has undergone a significant transformation, driven by the integration of machine learning (ML) and artificial intelligence (AI) techniques. The talk will revise this path from its origin till nowadays, by providing a comprehensive overview of the current state-of-the-art in applying ML to electron microscopy, highlighting the advancements and future directions in this rapidly evolving field. [1] ![]() The talk will go through traditional challenges in electron microscopy, such as data acquisition, processing, and interpretation, which have historically limited the technique’s efficiency and accuracy. By leveraging ML algorithms, researchers have developed innovative solutions to automate and enhance various aspects of electron microscopy, from image reconstruction and denoising to feature extraction and classification. Key developments discussed include the application of convolutional neural networks (CNNs) for high-resolution imaging, the use of generative adversarial networks (GANs) for realistic image synthesis, and the implementation of reinforcement learning for optimizing experimental parameters. I will also focus on spectroscopy and briefly go through tomography and techniques like compressive sensing that enable a more precise and multidimensional analysis of materials at the nanoscale. The talk will feature a set of practical guidelines for microscopists and materials scientists, offering insights into selecting and applying ML tools effectively, even for those without extensive ML expertise, as parallelly done in other scientific disciplines. Finally, I will go through recent examples of a successful application of ML, deep learning, computer vision or generally AI to analyse high resolution (scanning) transmission electron microscopy data and unveil new physics from materials, with special emphasis on quantum materials. [2] References: [1] Nanoscale Horiz., 2022, 7, 1427-1477 [2] Botifoll, M et al. Submitted/In arXiv soon! Amitrajit Mukherjee - Title TBCInhomogeneous and disordered atomic arrangement, and machinery/thermal drifts, often vary (micro)spectroscopy signals of sub-micron materials, albeit the (bulk) composition and experimental parameters are identical. Such diversity can be harnessed to measure material’s purity, unveiling several concealed features via statistical inspection of heterogeneous signals. However, this approach requires efficient categorization of a substantial number of data, which is currently encumbered by laborious calculations, computational hurdles, and manual intervention. We present a robust unsupervised machine learning module that automatically clusters real-time high-dimensional microscopy signals and perform class-wise power spectral density calculation. This workflow is explicitly tested for wide-field fluorescence image analysis of semiconductor nanocrystal thin-films, while also proves beneficial for scanning tunnelling spectroscopy of electron donor-acceptor (2D) self-assemblies. We additionally demonstrate the impact of data-preprocessing on the clustering efficiency. As a programmed interface, our methodology would enable fast big-data-analytics for contemporary (micro)spectroscopes to achieve instant material characterization, spanning diverse spectrum of interests. Alexandra Day -
Traditionally, materials discovery has been driven more by evidence and intuition than by systematic design. However, the advent of “big data” and an exponential increase in computational power have reshaped the landscape. Today, we use simulations, artificial intelligence (AI), and machine learning (ML) to predict materials characteristics, which dramatically accelerates the discovery of novel materials. For instance, combinatorial megalibraries, where millions of distinct nanoparticles are created on a single chip, have spurred the need for automated characterization tools. This talk will present an ML model specifically developed to perform real-time binary classification of grayscale high-angle annular dark-field images of nanoparticles sourced from these megalibraries. We elaborate on the computational challenges and our solutions, including managing memory constraints, optimizing training time, and utilizing Neural Architecture Search tools. The final model outperformed our expectations, achieving over 95% precision and a weighted F-score of more than 90% on our test data set.
|